I Found a Significant Indirect Effect, But My Total Effect Is Not Significant! What Do I Do?
- Amanda Montoya
- May 12
- 9 min read
This question comes up a lot—via email, in workshops, at conferences. You've run a mediation analysis and found a significant indirect effect, but your total effect isn't statistically significant. Now you're wondering: did I do something wrong? Can I trust this result? What does it even mean?
Let’s unpack what’s happening and how to interpret these results.
What's Going On?
At first glance, it might feel paradoxical: how can part of an effect (the indirect path) be statistically significant when the whole (the total effect) isn’t? But this scenario is more common—and more meaningful—than you might think.
There are two possibilities that might be occurring, and in this blog we will walk through both. One possibility is that an inferential error has been made: statistics are faulty and do not always make the correct decision, so this could account for the results. The other possibility is that at the population level there is an indirect effect but no total effect. Let's walk through each of these.
Inferential Error Possibilities
The key here lies in understanding error types and statistical power.
Type I Error (False Positive): It's possible your significant indirect effect is a fluke—a false positive. This would mean there is no total effect and there is no indirect effect. But...
Type II Error (False Negative): It’s also possible your total effect is real, but you didn’t have enough power to detect it.
Most people assume that indirect effects are harder to detect than total effects. This intuition is based on the fact that indirect effects are products of coefficients (a * b), which many assume leads to reduced power.
But this isn't always true.
Power of Indirect vs. Total Effects
Kenny & Judd (2014) tackled this question in their Psychological Science paper. They showed that when the total effect and indirect effect are equal in size and sign (i.e., c = ab), statistical power for the indirect effect can be higher than statistical power for the total effect. Kenny & Judd offer a great analogy: imagine an outfielder trying to throw a baseball to home plate. Rather than throwing it all the way in one go, they throw to a teammate halfway. The two shorter throws (like the a and b paths) are more effective than one long throw (the total effect, c).
This result is particularly insightful and intuitive in connection to the concept of "proximal" mediation, which is when the mediator is "too close" to either the focal predictor (X) or the outcome (Y). If the mediator is proximal to X it is more of a manipulation check, and will not help gain statistical power: like throwing the ball to someone who is very close to you. They still have to get the ball all the way to home plate. Similarly if the mediator is proximal to Y it is serving as a close proxy for the outcome and also does not gain statistical power: like throwing the ball to someone who is very close to home plate.
Ultimately what this means is that we may have more power to detect an indirect effect compared to a total effect, even when they are the exact same size! Especially when the mediator is right in the middle we're optimizing power for the indirect effect, and so we may be able to detect effects we wouldn't otherwise be able to without the mediation analysis.
No Inferential Errors: Just Competing Effects
While it is possible that an inferential error has been made, it is possible at the population level for the indirect effect to be non-zero, and the total effect to be zero.
Hayes (2009) gives a very clear example where the total effect of X on Y is not statistically significant, but there are two competing mediators: one with a positive indirect effect and one with a similarly sized negative indirect effect. In a case like this, if the direct effect is near zero, the total effect, which is the sum of the direct and indirect effects, can be very close to zero.
Rather than invalidating your model, a significant indirect alongside a non-significant total effect might actually be an opportunity to learn more.
Let me share an example from my time as a social psychologist. As an undergraduate I conducted a series of studies to examine if group-work assignments in computer science classes might increase women's interest in taking the class (Montoya, Master, Cheryan, 2020). The insight comes from goal-congruity theory which suggests that people pursue careers which meet their goals, women tend to prioritize communal goals (focus on caring for others and working with others), but computer science is often not perceived as very communal.
In the first study we ran, we randomized women to read a syllabi for a computer science class that had group work or individual work (X), and measured their perception that computer science fulfills communal goals (M) and their interest in the class (Y). We did not find a significant total effect! But we did find a significant indirect effect. So what does this mean?
O'Rourke & MacKinnon (2018) have a great paper on the insights that can emerge from studies where the intervention (in my case group work) doesn't show a significant total effect, but what indirect effects can tell us in these contexts. In particular, we hypothesized that group work would increase interest because it increased communal goals fulfillment. Thus when we see there is no total effect, we might assume that our proposed mechanism was also wrong, but in this case we would be incorrect. Indeed what we saw was that the mechanism was working as hypothesized, but that there was this mysterious direct effect that was negative, such that our positive indirect effect and negative direct effect balanced out into a non-significant total effect. This type of pattern is often called "competitive" mediation.
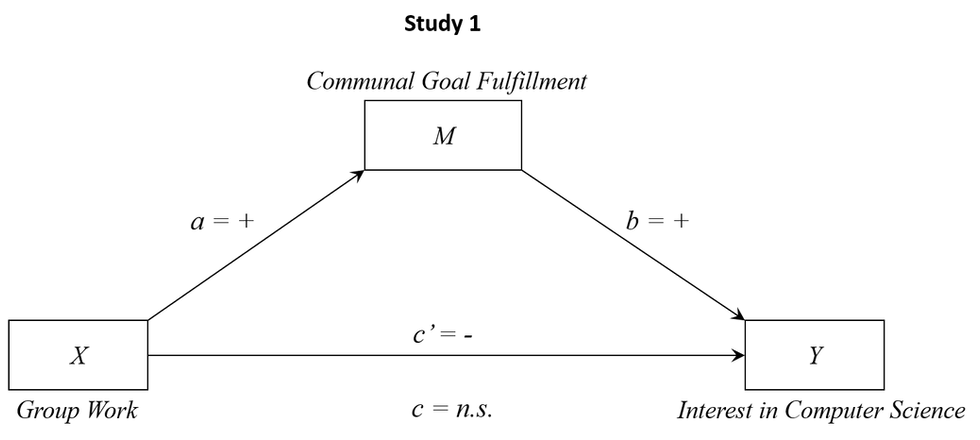
This led us to wonder if there might be some other mechanisms at play, independent of communal goal fulfillment. We began to think about what women might be thinking about group work that would dissuade them from taking a computer science class. One potential answer is stereotype threat: stress that occurs due to concerns about confirming a negative stereotype about one's group. Very reasonably, when a woman imagines working in a group in a computer science class, they might assume that there will be few women in the class and thus they will be the only woman in their group, eliciting stereotype threat. In a second study, we ran a similar procedure and measured stereotype threat, finding that it has a significant negative indirect effect, and communal goals again had a significant positive indirect effect.
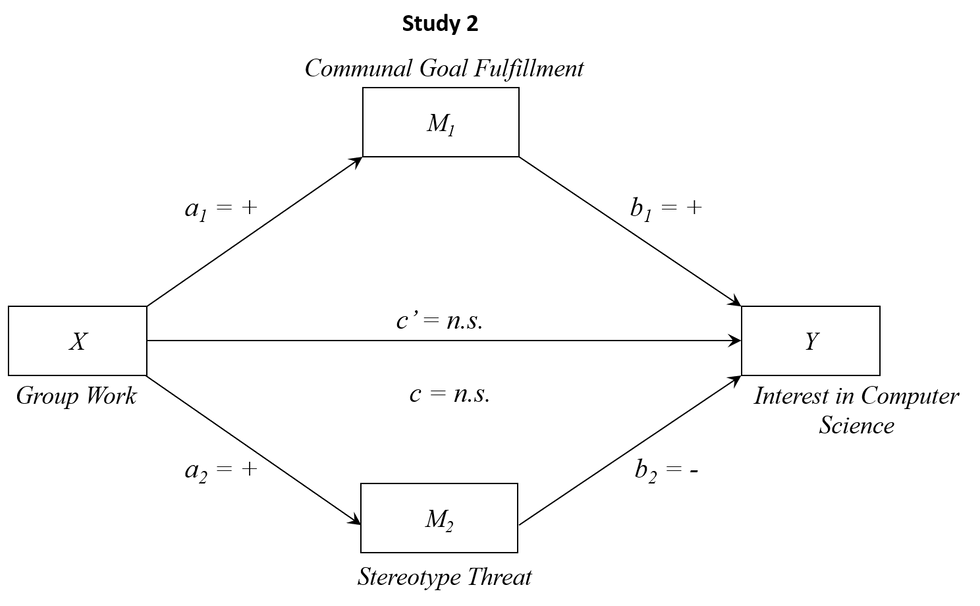
Next we thought about whether there might be some way to "turn off" the effect through stereotype threat. This would help us identify situations under which group work in computer science classes would increase interest for women. We ran our final study with a 2 (Group Work) x 2 (Numeric Representation) design, where the group work manipulation was the same as before. The numeric representation condition included informing participants that the class was typically 20% women or 50% women, and participants were randomly assigned to this condition. Again we measured communal goal fulfillment, stereotype threat, and interest in computer science.
What we found was very interesting: when women are underrepresented in the class or when no information was given, we essentially replicated the results from the previous study. We found no significant total effects, but a positive indirect effect through communal goal fulfillment and a negative indirect effect through stereotype threat. But, when gender representation was equal, the indirect effect through stereotype threat was no longer detectable. Ultimately, this resulted in the total effect of group work being significant in this condition (equal numeric representation).
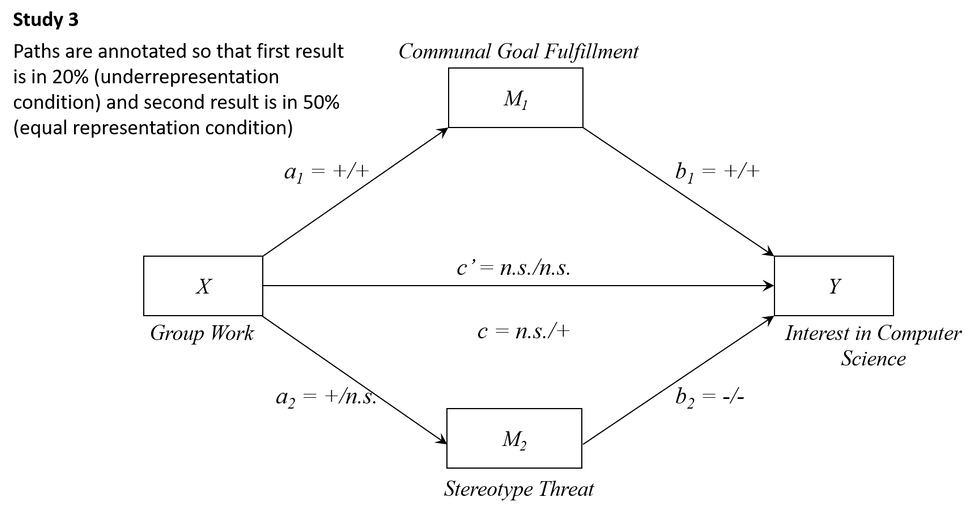
So what did we learn? Well, initially we thought that group work would help women get more interested in computer science, but the final insight is that this only works when there is gender parity in the class, so that group work does not induce stereotype threat.
This is a reminder: important insights can emerge from these seemingly conflicting results. Don’t toss them out just because the total effect isn’t significant. Get curious about what more you can learn!
A Quick Reality Check on Mediation Models
As we've just seen, non-significant total effects and significant indirect effects can co-occur for two reasons: 1) inferential error or 2) competing effects. However, I want to note here two issues that might also arise when thinking about these models. These problems are universal to mediation, not specific to the patterns focused on in this blog.
Is my model misspecified?
Many researchers become concerned when they see a non-significant total effect and significant indirect effect. Hopefully this blog has convinced you that these patterns of results can lead to deeper insights and important theoretical contributions, rather than something that indicates an error.
Of course, just because the indirect effect is significant doesn’t mean your mediation model is correct either. As is the case with any mediation model, we rely on a set of untestable assumptions to indicate whether the model is correctly specified (specifically no-confounding and temporal precedence).
But that’s always true—regardless of your results.
These kinds of “mixed” findings are not a special signal that your model is broken. They’re just one type of pattern that can emerge, especially in complex systems where multiple pathways are combined.
If the direct effect is zero, have I identified all the mediators?
Earlier I argued that a significant direct effect can indicate that there may be additional mediators that have not been explored in the model. The logical converse of this may seem to suggest that if the direct effect is zero, then there are no additional mediators. This is a situation often called "complete mediation." Many researchers have written against the use of this term, and that it does not mean what it sounds like. In particular, complete mediation is often interpreted to mean that we have identified all the mediators of the effect of X on Y. While this topic probably warrants a whole other blog, I just want to point out a specific counter example.
Consider an example like that in the Figure below, where there are 3 mediators of the effect of X on Y. M1 and M3 have a negative indirect effect and M2 has a positive indirect effect. Let's just assume all these indirect effects are of similar magnitude and the mediators are independent after controlling for X. In this case, Researcher 1 might measure M1 only, finding there is a significant positive indirect effect and no significant direct effect (which captures the indirect effects of M2 and M3), they might then conclude that M1 completely mediates the effect of X on Y. Researcher 2 conducts a similar study but only measures M3, finding that it too also completely mediates the effect of X on Y. How could both M1 and M3 completely explain the same effect? And in the end, neither has identified all 3 of the mediators that actually explain the effect of X on Y.
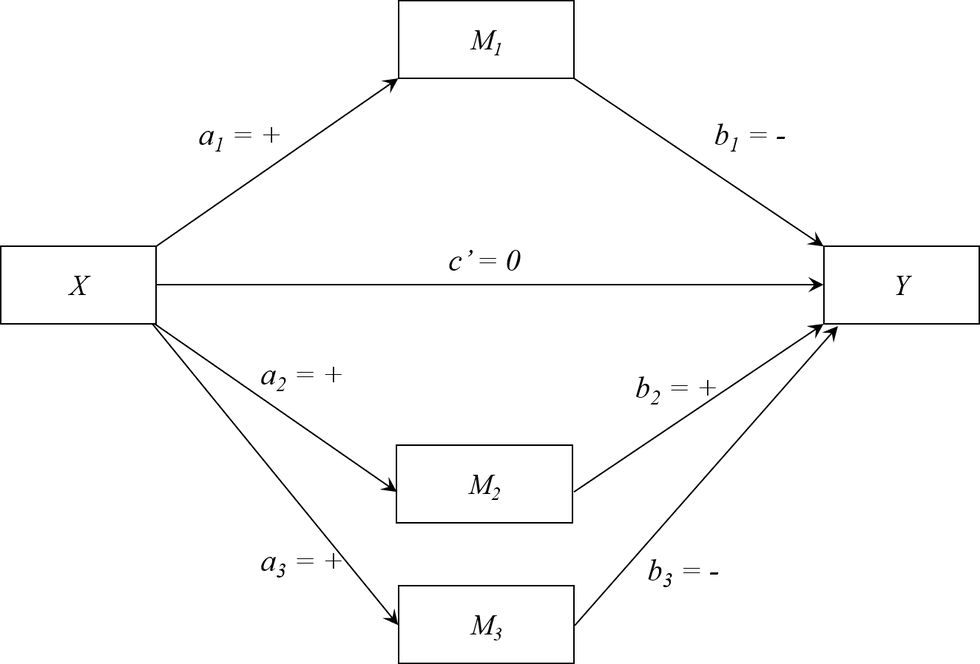
So while a significant direct effect can point to there being unidentified mediators, so too should a non-significant direct effect. First, we cannot accept the null hypothesis that the direct effect is zero, but also the direct effect is the sum of all unmodeled mediators, which could be a combination of positive and negative indirect effects.
Can I Publish This?
Yes. Many studies have been published with this pattern of findings. (If you have any examples, please share in the comments!)
But be careful about what claims you make.
One of the most common errors that researchers make is in accepting the null hypothesis about the total effect. I see this all the time in papers, where once you get to the conclusion section there are claims that are not supported by the statistical approach.
Don’t say your intervention “works” just because the mechanism worked. If your total effect isn’t significant, there’s no clear evidence that your manipulation changed the outcome overall.
Unfortunately, some published papers fall into this trap—especially in their conclusions. It’s easy to get excited and oversell a mediation pathway as if it stands in for a total effect. But reviewers and readers increasingly know to look out for this. Be transparent about your findings and cautious in your interpretations.
Instead of claiming success prematurely, use your results to generate new insights. This is your chance to uncover hidden dynamics, refine your model, or design better follow-up studies.
So... Can I Say X Affects Y?
If by “affects” you mean is there a causal pathway from X to Y, and your indirect effect is significant and your assumptions hold (e.g., no unmeasured confounding, correct temporal ordering), then technically yes—there is an effect.
But if you’re asking: Does manipulating X lead to a detectable change in Y overall?—your current data say, not yet. Think about the original study of group work: After that first study, if you had asked me "Can we use group work to increase women's interest in computer science?" The answer would be no! The manipulation was not effective at moving the outcome on average. However, after additional investigation we were able to identify the conditions under which group work does seem to increase women's interest in computer science.
So be precise in your language. That nuance matters, both scientifically and practically.
Final Thoughts
Finding a significant indirect effect without a significant total effect is not an error. It’s not even necessarily a problem. It’s an invitation—to think more deeply, ask better questions, and refine your understanding of how things work.
And yes—your study is still publishable. Just tell the story honestly, explore what might be going on, and avoid overclaiming.
Want to dig deeper? Below I've included a list of references that I've noted in this blog, that you might find particularly interesting and relevant to this topic.
References
Hayes, A. F. (2009). Beyond Baron and Kenny: Statistical Mediation Analysis in the New Millennium. Communication Monographs, 76(4), 408–420. https://doi.org/10.1080/03637750903310360
O’Rourke, H. P., & MacKinnon, D. P. (2018). Reasons for Testing Mediation in the Absence of an Intervention Effect: A Research Imperative in Prevention and Intervention Research. Journal of Studies on Alcohol and Drugs, 79(2), 171–181. https://doi.org/10.15288/jsad.2018.79.171
Kenny, D. A., & Judd, C. M. (2014). Power Anomalies in Testing Mediation. https://journals.sagepub.com/doi/10.1177/0956797613502676
Montoya, A. K., Master, A., & Cheryan, S. (2020, May 31). Increasing Interest in Computer Science through Group Work: A Goal Congruity Approach. https://doi.org/10.31234/osf.io/ahgfy
ChaptGPT was used for both text generation and editing of this blog. The prompt generation and the final product was edited by the author.
Kommentare